The Real Risk of AI in Engineering and Construction
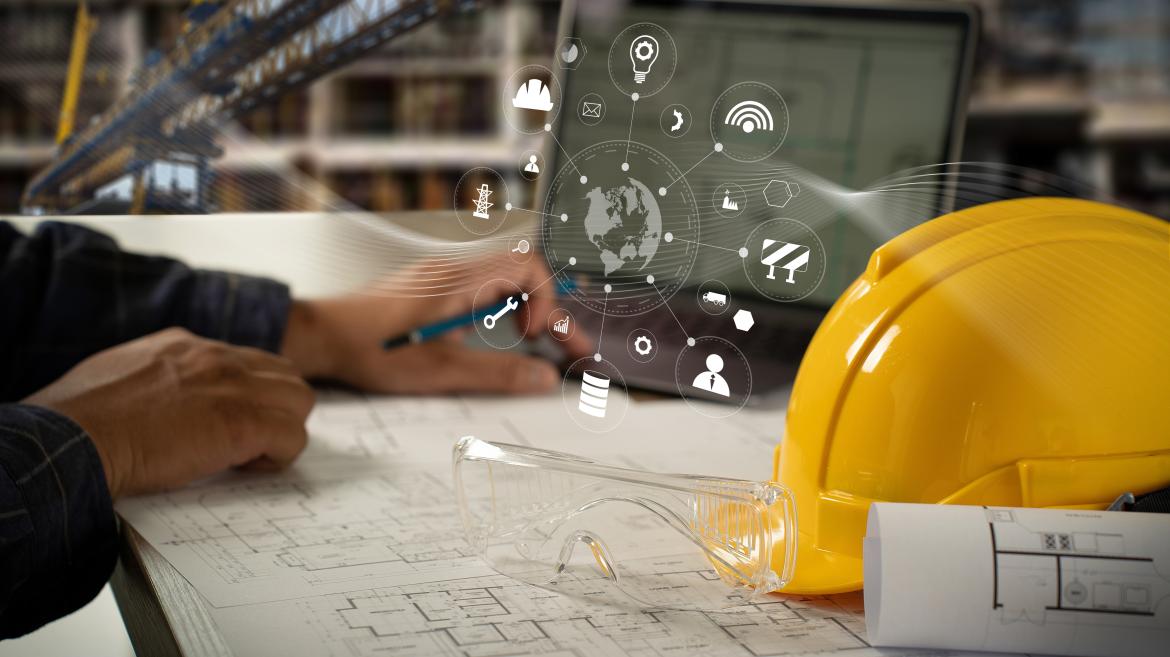
AI is here, and it’s not going away. It’s in the software we use to do our work and the helpdesk we might call if that software doesn’t work. It’s in the articles we read, the pictures and videos we see, and even the phone calls we receive. It’s in engineering and construction, and it’s not going away.
AI can make our work faster and more efficient. It might even make it better, but for now, it’s use is increasing the risk of major errors. When we work with other people, we form opinions about their level of competence. How much can they be trusted to work independently? What tasks can they do with supervision? What level of review does their work product require? The answers differ depending on who’s doing the work.
We also train humans to express their doubts and ask questions. Engineers are trained not to guess at solutions, bury those guesses deep in their calculations, and hope that a reviewer will find any mistake. They can ask questions up front, say when they’re uncertain, or highlight portions of work product for more detailed review. Likewise, reviewers learn to focus extra attention on critical parts of a design—those that are common sources of error or that might result in a severe failure. We rely on our training and processes for quality and ultimately for safety.
Today’s AI tools present a major challenge for our existing quality systems. AI tools produce work based on probability, not intuition, understanding, or experience. The tools work quickly, but there are few controls. We have examples of AI journalism citing nonexistent sources, legal briefs citing fake case law, and tax software giving bad advice. Humans make mistakes but few intentionally produce fake work. AI has no ability to understand whether the content it produces is real or fake. It’s just giving the most likely answer based on the query, its programming, and the many terabits of data it has digested. When reviewing AI work product, it’s important to remember that an AI design tool has zero ability to assess how anything it does relates to the real world.
“The work it takes to generate outcomes like text and videos has decreased, but the work to verify has significantly increased.”
Hatim Rahman, assistant professor at Northwestern University’s Kellogg School of Management, quoted by Danielle Abril, “I used AI work tools to do my job. Here’s how it went.” The Washington Post, February 26, 2024
Therein lies the great risk. We humans are not particularly well designed for spotting errors and inconsistencies in unexpected places. Our brains look for efficiency, allowing us to focus our attention on the things that we believe are most important. When we’re working with other humans, that can be helpful, but AI produces errors in new and unexpected ways that we’re not trained to spot. It can design things that no reasonable human would ever design. It can present unreasonable answers under a veneer of reasonableness that makes them harder to spot—like a three-armed person in the middle of a crowd.
There is no doubt that AI will be used in engineering and construction to generate bills of material and cable schedules, prepare purchase orders, and write safety and quality plans. Soon, it will design rebar cages, wire relays, and route pipe. How will human reviewers spot the errors? The same way we always have, by paying more attention to the most critical elements of the work. The challenge is that AI is going to make new errors that we’ve never seen before, and they will be in places that we don’t always look.
Humans are not particularly good at finding a needle in a haystack. As AI work product gets better, it may actually get harder to find serious errors as they are buried in what looks like thoughtful work product. To help manage the risks created by AI, we need to be transparent about when and how we are using it. We should evaluate tools by giving them erroneous inputs, faulty assumptions, and incomplete data. Then, we should apply multiple, independent human reviewers to trial work product, checking every detail—counting every rebar, stressing every pipe, and rewiring every termination. More than just assessing the AI, this process will teach us how to review AI work product, which will require different skills than reviewing human work product.
In engineering and construction, we are taught to share our doubts and ask questions. We can feel risk through our doubt and discomfort. We can take personal responsibility for our errors. AI can’t take responsibility. We can’t suspend its license or charge it with negligence. Humans are still the last line of defense, and we will be held accountable for the AI we use to help us.